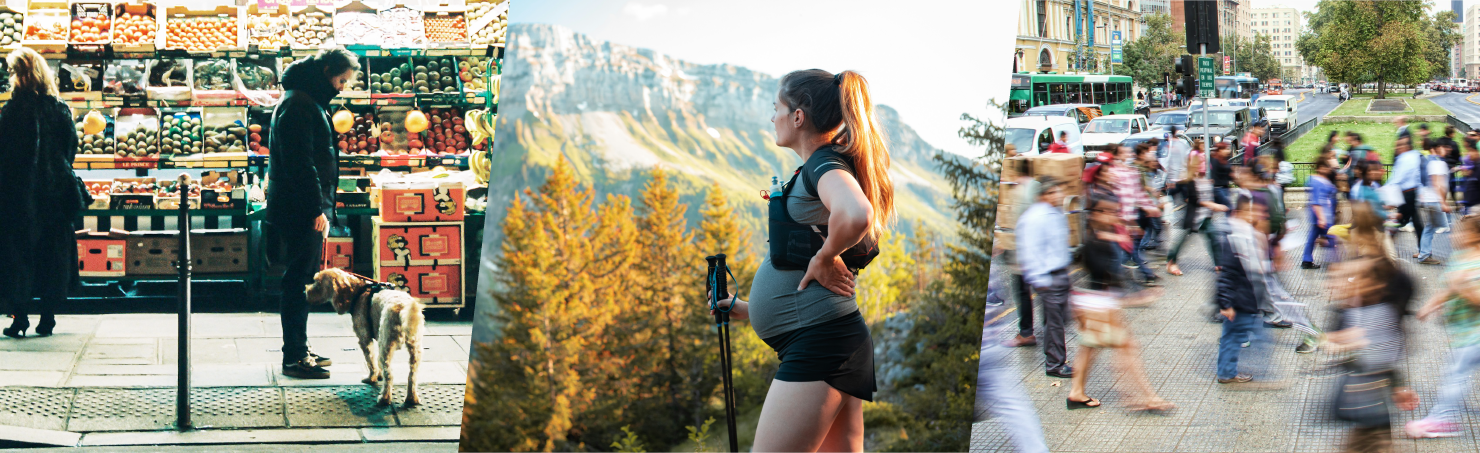
XPOSE
Doctoral Training in Exposome and Health
Navigating Complexity with Innovation
Axis 1: Assessment of environmental factors through cutting-edge methods
Lead: Dr Maria Ruiz-Castell (LIH/DoPH) & Prof. Emma Schymanski (UL/LCSB)
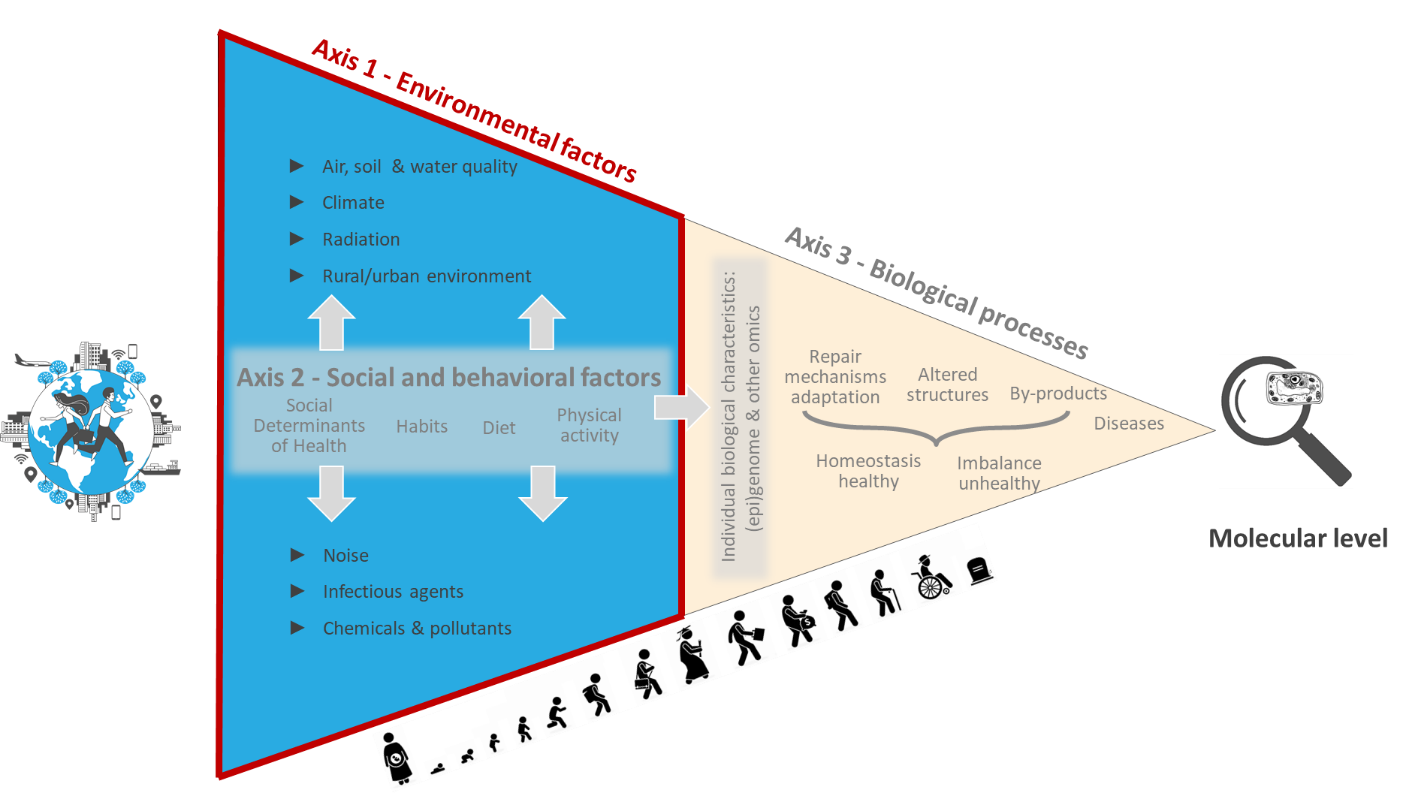
P1 – Establishing baseline exposures for Luxembourg adults using the EHES-Lux Study
Non-target high-resolution mass spectrometry (NT-HRMS) of patient samples holds great potential for revealing information about e.g., chemical exposures, medical conditions and current medications with one quick measurement. However, defining “baseline exposures” in a chemical space of >100 million chemicals is extremely challenging. This PhD project will use the NT-HRMS and open science cheminformatics approaches to characterize the typical adult exposome in Luxembourg, using samples from the Luxembourg adult population. The results will be matched with data available through questionnaires and targeted analysis of chemical exposure, to help interpret and validate the results.
The study will allow further consolidating open exposomics workflows and forming a baseline for future precision medicine efforts based on NT-HRMS and cheminformatics approaches.
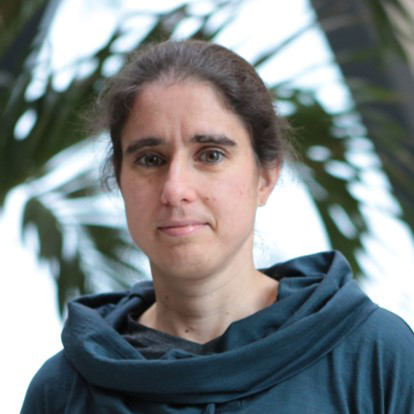
Schymanski
UL/LCSB
Supervisor
Profile
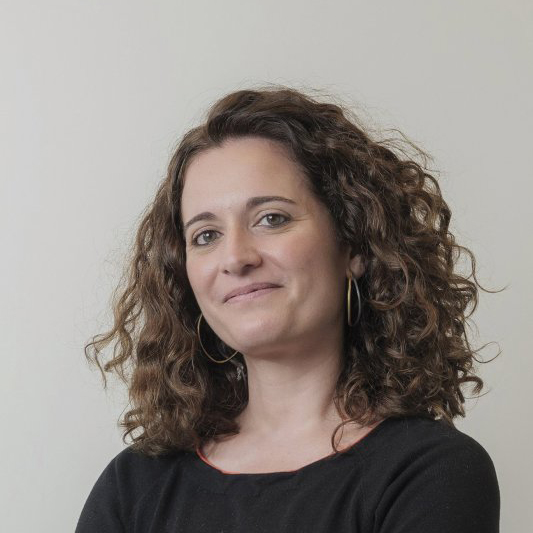
Ruiz
LIH/DoPH
Complementary thematic expert
Profile
P2 – Effect of the environmental factors on mental health in the general population using a multi-exposure approach
Mental disorders are among the top 10 global health burdens. While environmental exposures have been proven to impact depression, most studies have focused on single exposures. This PhD project will assess the combined effects of multiple environmental factors (e.g. air pollutants, noise and climate), using Geographical Information System (GIS) spatial analysis, on depressive symptoms in the Luxembourg population study and investigate differences by socioeconomic status. Exposure-wide association study (ExWAS) and deletion-substitution-addition (DSA) variable selection will be used for this multiexposure approach.
Understanding the role of the external exposome on depressive symptoms will help target public health strategies to address environmental conditions, reduce socioeconomic inequalities and improve mental health and quality of life.
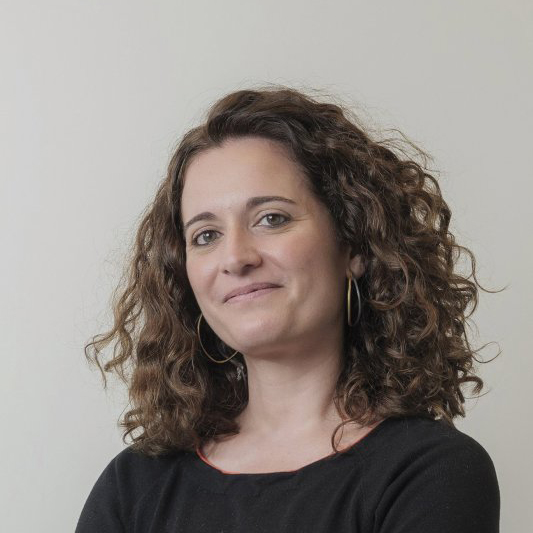
Ruiz
LIH/DoPH
Supervisor
Profile
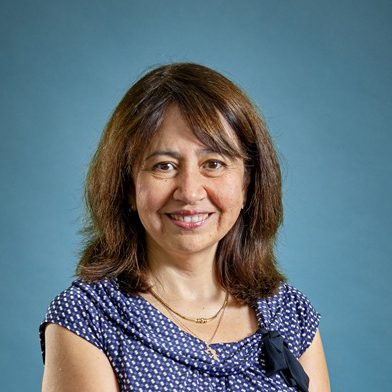
Aguayo
LIH/DoPH
Complementary thematic expert
Profile
P3 – Associations between exposomic phenotypes, glycemic variability and complications in people with type 1 diabetes
Glycemic variability (GV) is an important determinant of diabetes-related micro- and macrovascular complications and is a source of diabetes distress and poor quality of life in people with type 1 diabetes (T1D). Lifestyle factors are drivers of GV, but little is known on other potential risk factors. The PhD project will investigate the influence of environmental factors on GV and the risk of complications in people with type 1 diabetes.
Using data from the large SFDT1 cohort study augmented by innovative voice-based data collection, the PhD student will develop machine learning based approaches (including, but not restricted to, DDR-Tree and network analyses) to study the cocktail effect of multiple environmental factors (air quality, pollen, noise pollution, water quality, UV and weather) on diabetes-related outcomes. This will allow identifying exposomic phenotypes relevant in T1D care and defining personalised exposome-based interventions to prevent or delay diabetes-related complications.
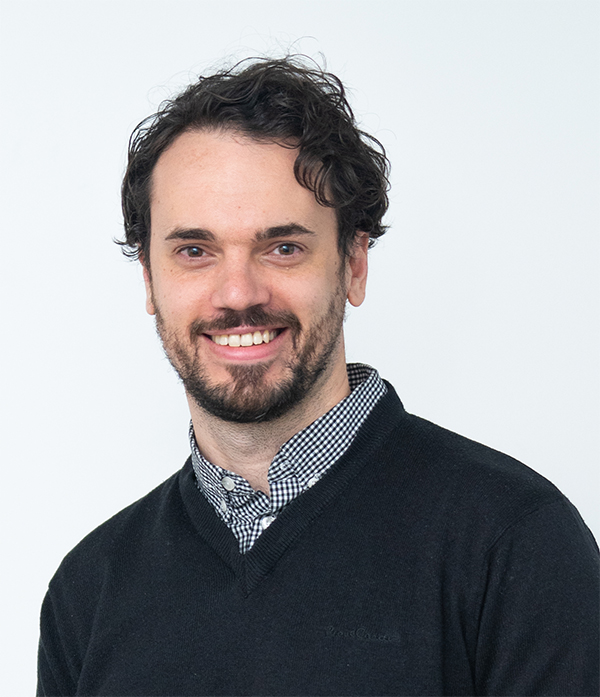
Fagherazzi
LIH/DoPH
Supervisor
Profile
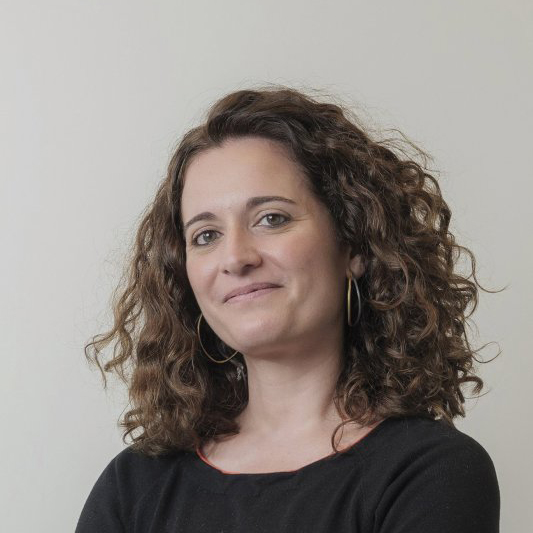
Ruiz
LIH/DoPH
Complementary thematic expert
Profile
P4 – Complex relationships between outdoor air pollution, social determinants of health, individual risk burden, and neurodegenerative and cardiovascular health outcomes
Outdoor air pollution has adverse effects on cognitive functioning and a range of neurodegenerative disorders as well as cardiovascular outcomes. Influence of sex/gender, socioeconomic status (neighbourhood deprivation, education level, income, occupation), and cardiovascular burden have so far not been systematically explored in this context. This PhD project will use computational analyses including applying machine learning approaches to analyse data of spatial air pollution information linked with social and health data of a big longitudinal population cohort together with the air pollution information.
Understanding possible effect modification of outdoor air pollution may help to identify vulnerable individuals at risk for adverse outcomes, enabling a precision-medicine approach.
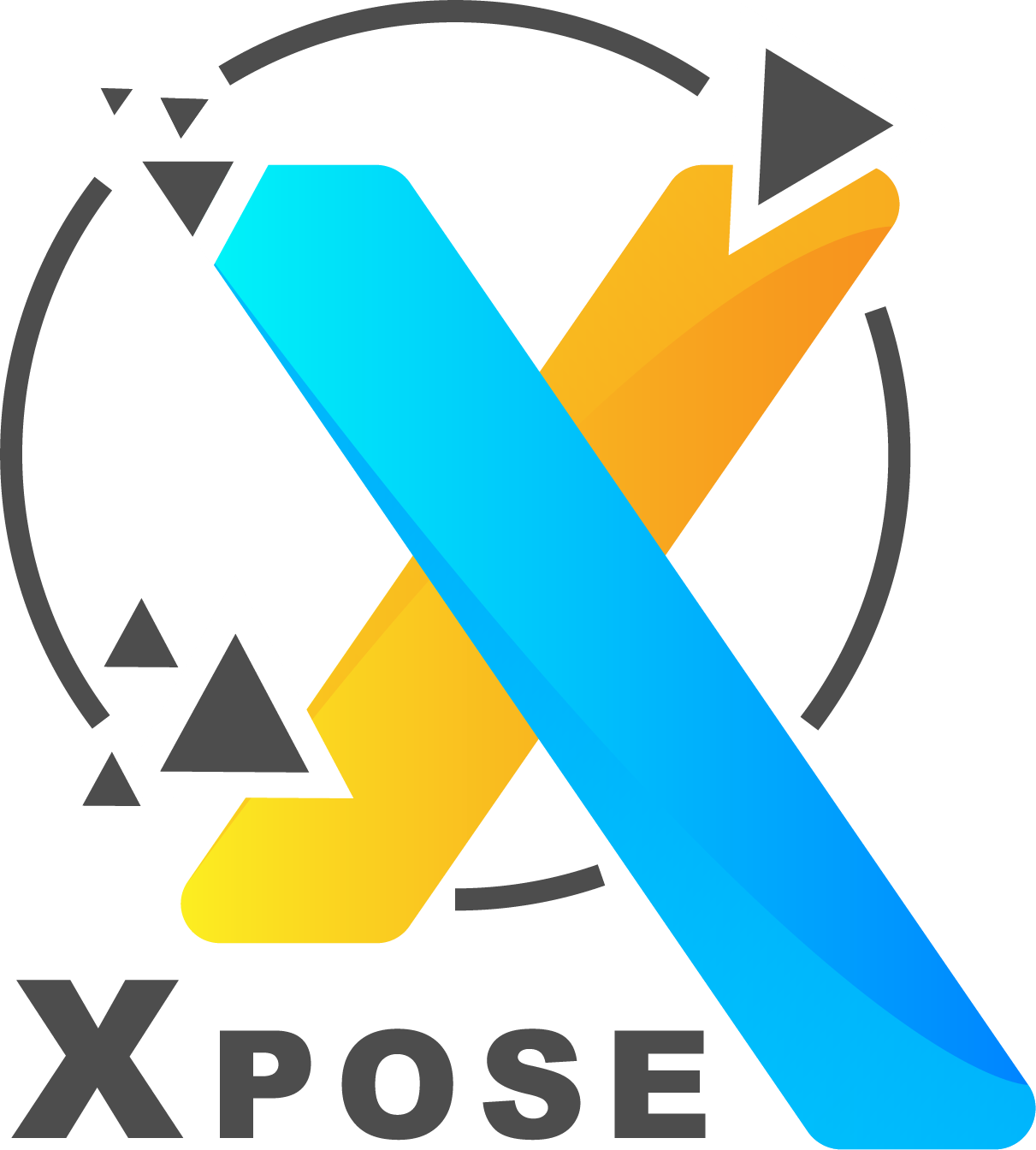
DISCOVER XPOSE
Exposome researcher of tomorrow
CONTACT
For any question related to XPOSE, please contact:
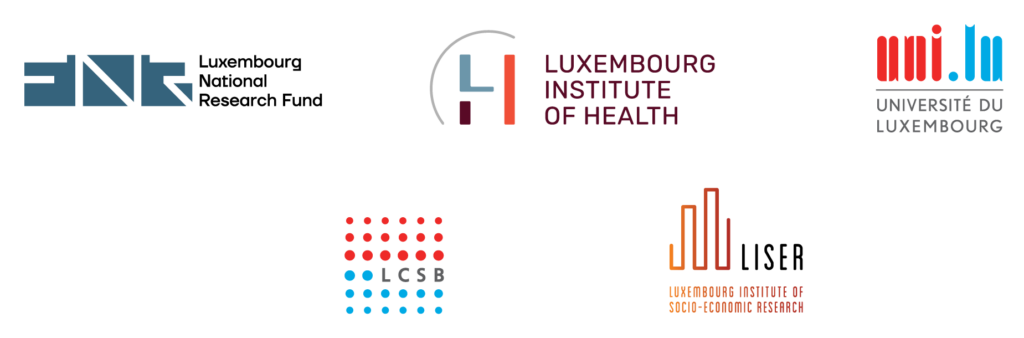
our open positions
We offer 12 fully funded PhD positions with a fixed-term work contract of up to 4 years.
We are seeking excellent and highly motivated candidates holding a Master’s degree in a field related to the topics of the doctoral training program. Very good English language skills are required. The earliest start date will be October 2024.